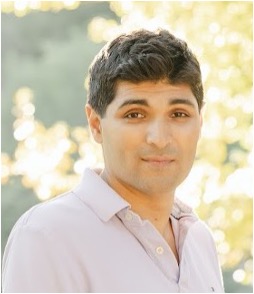
Norbert Wiener Professor of Mathematics, MIT
Director, Statistics and Data Science Center
Principal Investigator, Computer Science and Artificial Intelligence Laboratory
David and Lucile Packard Foundation Fellow
Alfred P. Sloan Foundation Fellow
Research
I am a theoretical computer scientist, and a major goal in my work is to give algorithms with provable guarantees for various problems in machine learning. See my publications and talks for more information. I am a member of the Theory of Computation group, MachineLearning@MIT, Foundations of Data Science and the Center for Statistics.
Book
If you are interested in recent developments in algorithms for machine learning, check out this book, which was published by Cambridge University Press.
Seminars
I co-organize the Theory of Computing Colloquium and the Harvard/MIT/MSR Reading Group.
Workshops
I co-organized New Challenges in Machine Learning: Robustness and Nonconvexity at STOC 2017.
I co-taught Learning at Scale, a summer school at MADALGO in 2014.
I co-organized Overcoming Intractability in Unsupervised Learning at STOC 2014.
I co-organized Topic Models: Computation, Application, and Evaluation at NIPS 2013.
Service
I was/will be on the Program Committee for FOCS 2023, STOC 2023, ITCS 2023, NeurIPS 2022 (area chair), COLT 2022 (area chair), ICLR 2022 (area chair), NeurIPS 2021 (area chair), COLT 2021 (area chair), ICLR 2021 (area chair), COLT 2020 (senior pc), NeurIPS 2019 (area chair), ICML 2019 (area chair), COLT 2019, NIPS 2018 (area chair), COLT 2018, ICALP 2018, STOC 2018, RANDOM 2017, SODA 2015, FOCS 2014 and APPROX 2013. I am also an Associate Editor for ACM Transactions on Algorithms.
Grants
My research is supported by a David and Lucile Packard Fellowship, an ONR Young Investigator Award, an NSF Large (with Barak, Kelner, Parrilo) CCF-1565235, an NSF CAREER Award, an Alfred P. Sloan Fellowship, a Microsoft Trustworthy AI Grant and a Google Research Award.
Contact
Email: "lastname" at mit dot edu
Offices: 2-472 (default) and 32-G594