The Value of Predictive Models
5/5/2009
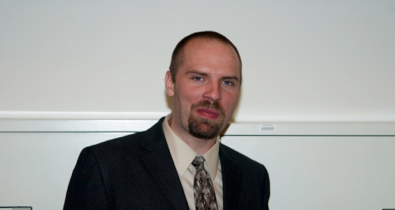
|
Caleb Hug defended his
thesis on April 30, 2009. His work brought me to
thoughts about the clinical utility of predictive models. |
Caleb Hug’s thesis, titled “Detecting Hazardous Intensive Care
Patient Episodes Using Real-time Mortality Models” develops a set
of models from the tens of thousands of intensive care unit
patients whose records are in the MIMIC II database. The purpose
of these models is to compute and evaluate a real-time varying
acuity score for each patient, which is in a sense a dynamic
indicator of how sick a patient is, and whether he is improving or
getting worse. Because we have no “gold standard” estimate
of acuity, instead he uses the likelihood of the patients’ dying
as a proxy for how sick they are.
Some of these models predict the risk of mortality within a month,
using a large variety of objective data recorded about the patient,
including lab values, instrument settings, intravenous infusions,
etc. Among these mortality models, some are based on aggregate
data from the patient’s multiple days of stay in the ICU, some
depend on data on each specific ICU day, and one relies on the
dynamically changing data and is re-calculated every time new
relevant data are measured. He also computed an approximation to the
commonly-used SAPS II score as a point of comparison. Each of his
acuity models, roughly independent of the duration of time they were
trained on, succeeded about equally well in its prediction tasks, as
measured by area under the ROC curve on an independent test set of
cases. And they all performed better than his approximation to
the SAPS II score, based on our data. This is good news for
the overall goal, because it shows that the real-time model does
accurately track the likelihood of eventual outcome for the patient,
and therefore appears to be a good proxy for how sick the patient
is.
In addition to the mortality models, Caleb also developed a number
of more focused models that predict specific events to happen within
the next several hours. These include weaning from administration of
vasopressors, weaning from an intra-aortic balloon pump, development
from infection to septic shock, and acute kidney injury. Somewhat as
we expected, these specialized models make more accurate predictions
for their target conditions than what one can obtain from any of the
mortality models. This suggests that with sufficient experiential
data it is possible to make reasonably accurate predictions about
when either good or bad events can be anticipated during a patient’s
ICU stay.
After Caleb presented the same results to our Biomedical Research
Partnership (BRP) group today, Roger Mark, the PI of this project,
and I got into an interesting discussion about the value of
predictive models, how one can go about evaluating them, and how (or
whether) to put them into clinical use. This led me to the
following thoughts:
- Even if we had a perfect predictive model (i.e., 100%
sensitive and specific), how would we use it. For example,
suppose that we could develop a model that examines the health
state of an ICU patient and tells us, with absolute accuracy,
whether that patient would survive his stay in the ICU and for
at least the next month thereafter. What would be the use
of such a model? If patient Smith were predicted to die,
would we therefore immediately “pull the plug” on him? If
patient Jones were predicted to live, would that mean we could
pay less attention to him? Ultimately, these scenarios are
unappealing and not realistic. It’s impossible to make 100%
accurate predictions, in part because the true outcome depends
on the behavior of clinicians who will be influenced by those
predictions.
- So what about an imperfect prediction? For much shorter
term uses, such as alarms sounded based on physiological
measures, we generally think that some combination of
sufficiently high sensitivity and specificity, even if
considerably short of 100%, are acceptable. For example, an
asystole alarm may be excused even if its positive predictive
value is only 20% so long as it is highly sensitive. This is
because the costs of a false positive and a false negative are
dramatically different. In the first case, nurses get annoyed by
a spurious alarm, whereas in the second, the patient may die
unnoticed. Nevertheless, in practice we hear of ICU nurses
turning off important alarms just because the cacophony of
frequent alarm sounds overwhelms their ability to respond
sensibly.
- In the case of much longer-term predictions such as a high
likelihood that a patient will not survive for the next 30 days,
it’s hard to know how clinicians will or should respond even if
the prediction is reasonably accurate. It seems reasonable
to assume that they would devote extra attention to the case, be
more aggressive in treatment, or try some alternative approach
if warned that the current path is downhill. However, it’s
hard to know whether that extra attention or change of direction
can be sustained over such a long duration as that of the
prediction. These predictions don’t have the immediacy of
the asystole alarm.
- Roger suggests that, despite the predictive model’s roots in
mortality prediction, we should think of it instead as what we
mean it to be, namely a dynamically changing needle showing the
current aggregate health state of the patient. Caleb also
suggests that it is really a measure of how this patient is
doing, based on a comparison to previous patients in similar
situations. This seems like a more sustainable view, but
itself raises two questions:
- I don’t know how to create a gold standard for health
state. So, we build and calibrate the model on the task of
predicting mortality, but then use it as a measure of health
state. This is certainly our approach, but is it legitimate?
- We can certainly provide such a measure as a clinical
indicator to ICU clinicians and then see whether it alters
their behavior. If yes, then we can see if it leads to better
outcomes for their patients. Again, if yes, then it is
clearly a useful intervention. Is there a simpler way to
assess this hypothesis? Roger suggests asking ICU staff
to rate patients’ health state on a subjective scale, and then
studying the degree to which Caleb’s acuity score correlates
with this. I suspect that this is the most practical
current approach.
Back to Blog
Accessibility