Medical
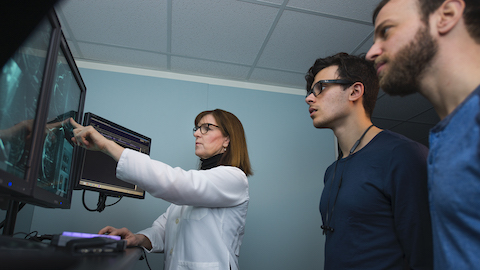
Today, the vast majority of breast cancers are diagnosed from mammograms. While radiologists are skilled in identifying suspicious areas once cancer is already visible on the image, they have limited capacity in predicting which patients are heading towards cancer diagnosis before mass is formed. Reliably identifying such patients is the crucial first step in preventing cancer development. Substantial research in medical literature confirms that certain changes in the breast tissue are early precursors of cancer development. However, patterns identified by humans are not sufficiently strong to act upon them.
We are aiming to automate these predictions by developing deep learning algorithms that can using longitudinal imaging data with known outcomes. By reading millions of images (orders of magnitude more than humans can read in their lifetime), machines should be able to assess the likelihood of cancer occurrence in a more accurate fashion. We are currently collecting a large corpus that will enable us to answer this question. Meanwhile, we are building algorithms that can help radiologists with their daily tasks of mammogram reading. The deep learning models we developed today can accurately predict breast density and identify with human accuracy a large fraction of safe screening mammograms.