Burst Image Deblurring Using Permutation Invariant Convolutional Neural Networks
Miika Aittala and Frédo Durand, MIT CSAIL
ECCV 2018 (oral)
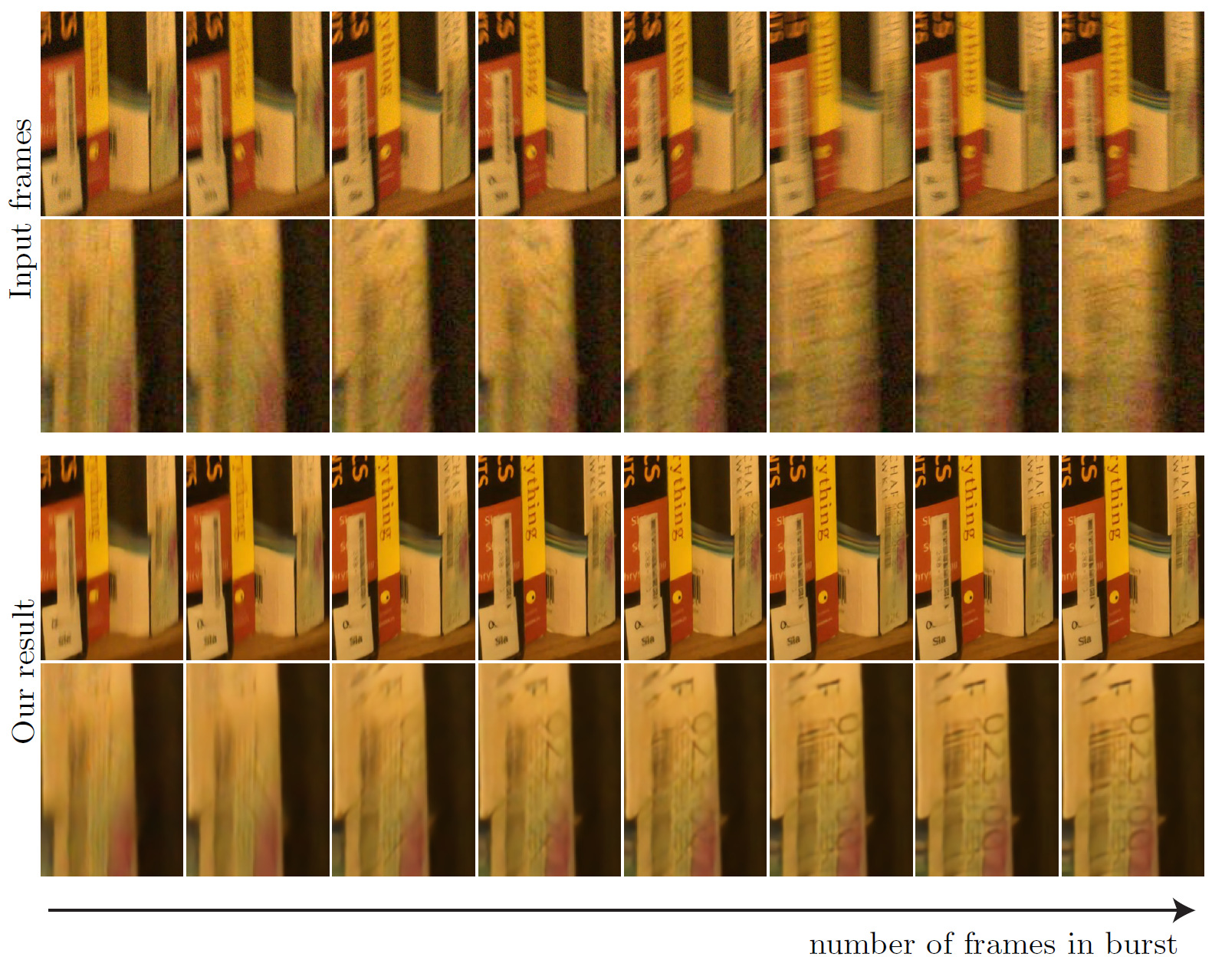
Abstract
We propose a neural approach for fusing an arbitrary-length burst of photographs suffering from severe camera shake and noise into a sharp and noise-free image. Our novel convolutional architecture has a simultaneous view of all frames in the burst, and by construction treats them in an order-independent manner. This enables it to effectively detect and leverage subtle cues scattered across different frames, while ensuring that each frame gets a full and equal consideration regardless of its position in the sequence. We train the network with richly varied synthetic data consisting of camera shake, realistic noise, and other common imaging defects. The method demonstrates consistent state of the art burst image restoration performance for highly degraded sequences of real-world images, and extracts accurate detail that is not discernible from any of the individual frames in isolation.
Publication
Miika Aittala, Frédo Durand
Burst Image Deblurring Using Permutation Invariant Convolutional Neural Networks
proceedings of European Conference on Computer Vision 2018
Downloads
- Paper preprint
- Supplemental appendix document
- Supplemental material package
NOTE: The images in this archive are highly compressed and are only intended for visual inspection. Please see the readme file within the archive. - Presentation slides at ECCV 2018 (PowerPoint)
- Datasets
- We are working on a cleaned-up version of the source code. In the meantime, please find a coarse version of the source code and a pre-trained model HERE (updated Oct 2020). Be sure to read the README.txt file.